Revolutionizing Software Development with Automatic Image Annotation
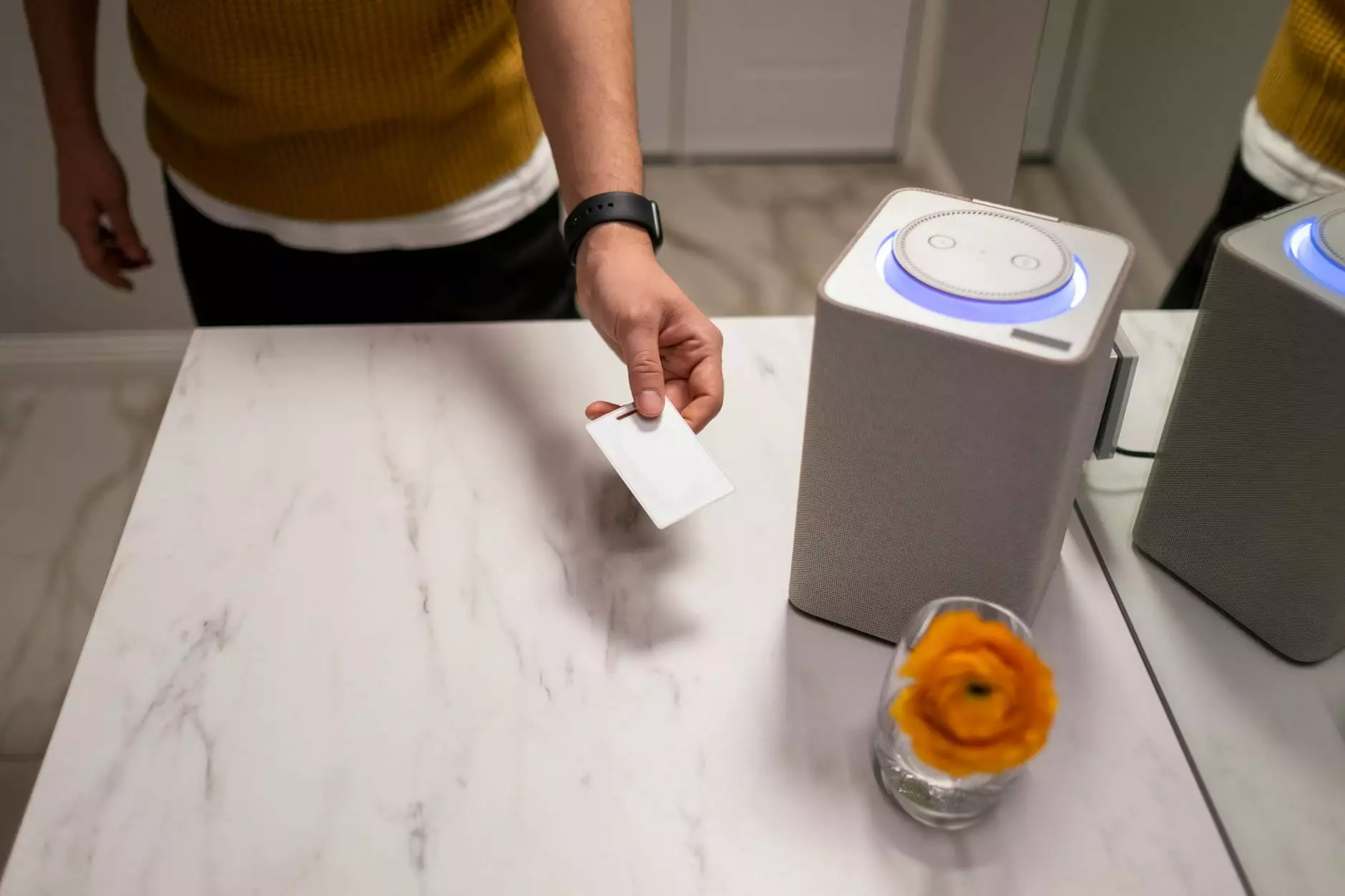
The digital landscape is continuously evolving, pushing businesses to innovate and adapt. One of the most exciting advancements in this evolution is automatic image annotation, a game-changer for software development and various other fields. In this article, we delve deep into the advantages, methods, challenges, and future prospects of automatic image annotation, showcasing its significant impact on enhancing the technological prowess of businesses like Keymakr.
Understanding Automatic Image Annotation
Automatic image annotation refers to the process of using algorithms to automatically label content in images. This technology understands the context and elements within the images, making it easier for machines to interpret visual data. This is incredibly valuable in industries that rely on visual content, such as retail, healthcare, and automotive.
The Importance of Image Annotation in Software Development
In software development, particularly in machine learning and artificial intelligence applications, image annotation plays a crucial role. Annotated images serve as training data for models that need to learn the features and characteristics of different objects. With automatic image annotation, developers can significantly reduce the time and effort required to prepare datasets.
Benefits of Automatic Image Annotation
Automatic image annotation offers a plethora of benefits to businesses, particularly those engaged in software development. Here are some key advantages:
- Efficiency: Traditional image annotation is a time-consuming task that requires human labor. With automation, this process is streamlined, allowing businesses to save valuable time.
- Cost-Effectiveness: By reducing the need for extensive manual annotation, companies can lower operational costs associated with data preparation and focus their budgets on core development activities.
- Scalability: As the amount of visual data continues to grow, manual annotation becomes impractical. Automated systems can easily scale to handle vast amounts of image data without compromising quality.
- Precision and Consistency: Manual annotation is susceptible to human error. Automatic systems provide consistent annotations, minimizing inaccuracies and biases in the data.
- Improved Data Utilization: High-quality annotated data leads to better-performing machine learning models, which are crucial for enhancing the functionality of software applications.
How Automatic Image Annotation Works
The mechanism behind automatic image annotation typically involves several advanced technologies, including:
1. Machine Learning Algorithms
Machine learning models, especially deep learning architectures like Convolutional Neural Networks (CNNs), play a pivotal role in image recognition. These models are trained on labeled datasets to understand various features and patterns within images.
2. Computer Vision Techniques
Computer vision forms the backbone of automatic image annotation. Techniques such as image segmentation, feature extraction, and object detection enable machines to analyze and understand the content of images effectively.
3. Natural Language Processing (NLP)
NLP algorithms can be employed to generate textual descriptions of image content. This can enhance the annotation process by providing contextual relationships between objects in an image.
Applications of Automatic Image Annotation
The implications of automatic image annotation span across various sectors:
1. E-Commerce
In the e-commerce industry, automatic image annotation can enhance product search and recommendation systems. By tagging images with relevant attributes, customers can find products more easily and receive personalized suggestions.
2. Healthcare
In the medical field, accurately annotating images such as X-rays or MRIs is critical. Automatic image annotation can assist in the rapid identification of anomalies and streamline the diagnostic process for healthcare professionals.
3. Autonomous Vehicles
Automatic image annotation is essential for training self-driving cars to recognize objects on the road, including pedestrians, traffic lights, and other vehicles. This technology drastically improves the safety and efficiency of autonomous driving systems.
4. Social Media
Social media platforms utilize automatic image annotation to enhance user experience through tagging and content organization. This enables better content discovery and targeted advertising.
Challenges in Automatic Image Annotation
While the benefits are substantial, there are challenges associated with automatic image annotation. Some of these include:
- Data Quality: The accuracy of annotations heavily depends on the quality of the training data. Poorly annotated data can lead to ineffective models.
- Complexity of Context: Understanding the context and nuances of images can be challenging, especially when images contain multiple objects or require subjective interpretation.
- Resource Intensive: Training models for automatic annotation can require extensive computational resources and expertise in machine learning.
- Bias in Algorithms: If the training data lacks diversity, it can result in biased annotations that do not generalize well in real-world scenarios.
The Future of Automatic Image Annotation
The future of automatic image annotation looks promising, with continued advancements in AI and machine learning likely to enhance its capabilities. Here are a few trends to watch:
1. Improved Deep Learning Models
As deep learning techniques continue to evolve, we can expect models to become even more adept at understanding complex visual information, resulting in higher accuracy in annotations.
2. Integration with Augmented Reality (AR)
Combining automatic image annotation with AR could revolutionize how users interact with visual content. This integration could provide real-time annotations and insights within physical environments.
3. Enhanced User Customization
Future systems may offer businesses and users more control over the annotation process, allowing for tailored annotations that meet specific needs and preferences.
4. Ethical Considerations
As with any technology, ethical considerations will play a significant role in shaping the future of automatic image annotation. Ensuring fairness, transparency, and accountability in algorithms will be paramount.
Conclusion
Automatic image annotation is set to transform software development and many industries by enhancing efficiency, accuracy, and user experience. Businesses like Keymakr stand to gain significantly from adopting such cutting-edge technologies. By embracing automatic image annotation, companies can improve their operations, provide better products and services, and stay ahead in a highly competitive market.
As the demand for visual data continues to grow, the role of automatic image annotation will become increasingly crucial. Its potential to streamline processes, reduce costs, and improve data quality makes it an invaluable tool for modern businesses. Staying informed and implementing these advancements will be key to thriving in the digital age.