Unlocking the Power of Data Annotation with KeyLabs.ai: The Ultimate Guide to Data Annotation Tools & Platforms
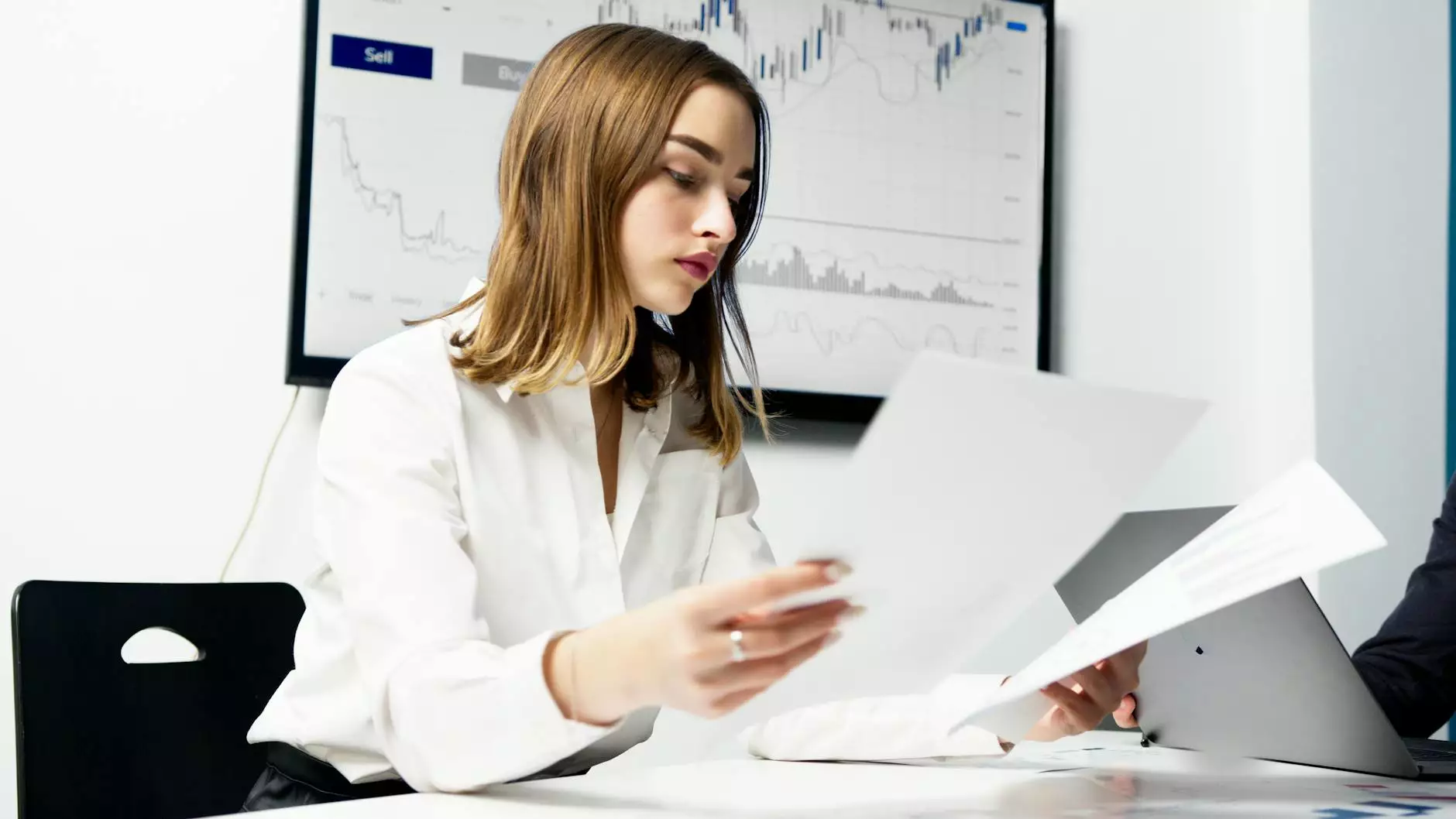
In the rapidly evolving world of artificial intelligence (AI) and machine learning (ML), the quality of data is the foundation upon which successful models are built. Whether you're developing computer vision applications, natural language processing tools, or autonomous systems, precise data annotation is critical to achieving high accuracy and robust performance. Among the myriad of data annotation techniques, bounding boxes stand out as one of the most widely used and effective methods, especially for object detection tasks.
Understanding the Role of Data Annotation in AI Development
At the core of AI development is supervised learning — a process where models learn from labeled datasets. Without accurate annotations, models cannot interpret the data correctly, leading to inaccurate predictions or classifications. This is where specialized data annotation tools come into play, streamlining the process and ensuring consistency across large datasets.
KeyLabs.ai provides state-of-the-art data annotation platform solutions, specifically designed to enhance efficiency, accuracy, and scalability in data labeling tasks. Its versatile data annotation tool leverages cutting-edge technology to meet the diverse needs of AI developers and data scientists.
Why Data Annotation is Critical for Machine Learning Success
- Data Quality: Accurate annotations directly influence model performance, reducing errors and biases.
- Efficiency: Automating parts of the annotation process saves time and reduces labor costs.
- Scalability: Robust platforms handle vast datasets, ensuring projects scale smoothly as data volume grows.
- Consistency: Standardized annotation protocols minimize discrepancies across annotators.
- Versatility: Supports multiple annotation types like bounding boxes, polygons, semantic segmentation, and more.
Deep Dive into Bounding Boxes: The Pillar of Object Detection
Among the various annotation techniques, bounding boxes are fundamental in training models for object detection tasks. They involve drawing rectangular frames around objects within images, allowing models to learn spatial relationships and object localization with high precision.
What Are Bounding Boxes?
Bounding boxes are simple, yet powerful, annotations consisting of four coordinates: x-min, y-min, x-max, and y-max. They directly enclose objects, creating a rectangular boundary that identifies the object's location within the image or video frame.
Applications of Bounding Boxes in AI
- Autonomous Vehicles: Detect pedestrians, vehicles, cyclists, and road signs.
- Retail and Inventory Management: Recognize products on shelves.
- Security and Surveillance: Monitor intrusions or suspicious activities.
- Medical Imaging: Locate tumors or abnormalities in scans.
- Robotics: Enable robots to recognize and interact with objects accurately.
Designing an Effective Data Annotation Platform with KeyLabs.ai
KeyLabs.ai's data annotation platform offers comprehensive solutions tailored to meet the demands of complex projects. Here are some of the critical features that make it a standout choice:
1. User-Friendly Interface
Streamlined, intuitive tools empower annotators to label data efficiently, reducing training time and minimizing errors.
2. Support for Multiple Annotation Types
Beyond bounding boxes, the platform supports polygons, semantic segmentation, line annotations, and more, accommodating various AI use cases.
3. Automated and Semi-Automated Tools
Integration of AI-assisted annotations accelerates workflows, ensuring high-quality labels with less manual effort.
4. Quality Control and Review
Built-in validation mechanisms ensure annotation accuracy, with options for multi-level review and consensus building among annotators.
5. Scalability & Collaboration
Designed for large datasets, the platform enables teams to collaborate seamlessly, assign tasks, and monitor progress in real-time.
Best Practices for Creating Accurate Bounding Box Annotations
To harness the full potential of bounding boxes, it’s essential to follow established best practices:
- Consistency in Annotation: Use standardized guidelines to maintain uniformity across annotators.
- Precise Object Boundaries: Enclose objects tightly without excessive whitespace to improve model learning.
- Multiple Annotator Review: Incorporate quality control checks to identify and correct discrepancies.
- Leverage Automation: Use AI-assisted tools, where available, to pre-label data for faster annotation cycles.
- Clear Definition of Object Classes: Ensure that each label is well defined and understood by annotators to prevent misclassification.
Challenges in Data Annotation & How KeyLabs.ai Overcomes Them
Data annotation, especially with complex datasets, can present several hurdles such as inconsistency, scalability, and time-consuming processes. KeyLabs.ai addresses these challenges through:
- Advanced Automation: Integrate machine learning models to assist and validate annotations.
- Robust User Management: Facilitate team collaboration with role-based access controls.
- Customizable Workflows: Adapt the platform to specific project needs, ensuring maximum efficiency.
- Data Security: Ensure that sensitive data remains protected with secure infrastructure and compliance measures.
Future Trends in Data Annotation and AI Readiness
The landscape of data annotation is constantly evolving, driven by advancements in AI and increasing data needs. Key trends include:
- AI-Augmented Annotations: Increasing integration of AI to pre-label data, leaving human annotators to verify and refine labels.
- 3D Annotations: Growing demand for annotations in 3D environments for AR/VR and robotics.
- Cross-Modal Labeling: Combining visual, textual, and audio data annotations for multi-sensory AI systems.
- Blockchain for Data Provenance: Ensuring transparent, tamper-proof data labeling processes.
- Enhanced Collaboration Tools: Real-time collaborative annotation interfaces to boost productivity and accuracy.
Choosing the Right Data Annotation Partner: Why KeyLabs.ai is Your Best Choice
Partnering with a reliable data annotation platform can significantly influence your AI project’s success. KeyLabs.ai distinguishes itself through:
- Unmatched Precision: Prioritizing accuracy to ensure high-quality labeled data.
- Scalability: Handling large datasets without compromising performance or quality.
- Customization: Tailoring annotation workflows to meet specific project requirements.
- Expert Support: Dedicated customer service and technical assistance throughout your project lifecycle.
- Cost-Effectiveness: Delivering premium annotation services at competitive prices.
Final Thoughts: The Future of Business and AI with Accurate Data Annotation
The rapid growth of AI-driven applications across industries underscores the importance of precise data annotation—especially techniques like bounding boxes—to unlock the true potential of intelligent systems. By leveraging advanced data annotation tools and platforms like KeyLabs.ai, businesses can accelerate their AI projects, improve model accuracy, and achieve a competitive edge.
Embracing innovative annotation solutions is not just a technological upgrade; it’s a strategic investment that can transform data into actionable insights, streamline workflows, and foster innovation across sectors. As AI continues to evolve, so will the tools and techniques for data labeling, making expert platforms like KeyLabs.ai indispensable partners in your digital transformation journey.
Invest in high-quality data annotation today to future-proof your AI initiatives and push the boundaries of what your business can achieve.